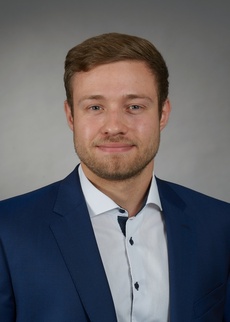
Marco Wurster, M.Sc.
- Research Associate
- department: Production Systems
- office hours: to be agreed
- room: 104, Geb. 50.36
- phone: +49 1523 9502599
- Marco Wurster ∂does-not-exist.kit edu
76131 Karlsruhe
Kaiserstraße 12
Marco Wurster, M.Sc.
Area of Research:
- Machine Learning for production planning and control
- Scalable automation of agile production systems
- Plug&Produce
- Remanufacturing at factory level
- Factory planning
General Tasks:
- Coordination of lecture "Integrated production planning in the age of Industry 4.0" (IPP)
- Assembly planning: concept and detailed planning
- Coordinator Learning Factory Module Scalable Automation
- Representative in the IHK Karlsruhe "Arbeitskreis Industrie 4.0"
Projects:
Test benches:
Curriculum Vitae:
sincet 10/2019 | Research Associate at the Institute of Production Science (wbk) at Karlsruhe Institute of Technology (KIT) |
10/2012-06/2019 | Study of Mechanical Engineering at Karlsruhe Institute of Technology (KIT) |
09/04/1993 | Born in Breisach am Rhein |
Publications
The Bio-inspired Changeable Production System – A Stem Cell Approach
Gartner, P.; Bilger, M.; Wurster, M.; Kandler, M.; May, M.; Lanza, G.
2024. Procedia CIRP, 130, 1256 – 1261. doi:10.1016/j.procir.2024.10.236
Gartner, P.; Bilger, M.; Wurster, M.; Kandler, M.; May, M.; Lanza, G.
2024. Procedia CIRP, 130, 1256 – 1261. doi:10.1016/j.procir.2024.10.236
Adaptive Multi-Priority Rule Approach To Control Agile Disassembly Systems In Remanufacturing
Wurster, M.; Bail, F.; Behrendt, S.; Lanza, G.
2023. Proceedings of the 5th Conference on Production Systems and Logistics (CPSL-2). Ed.: D. Herberger, 782–792, publish-Ing. doi:10.15488/15278
Wurster, M.; Bail, F.; Behrendt, S.; Lanza, G.
2023. Proceedings of the 5th Conference on Production Systems and Logistics (CPSL-2). Ed.: D. Herberger, 782–792, publish-Ing. doi:10.15488/15278
Interoperable Architecture For Logical Reconfigurations Of Modular Production Systems
Behrendt, S.; Wurster, M.; Strljic, M.; Klein, J.-F.; May, M. C.; Lanza, G.
2023. Proceedings of the 5th Conference on Production Systems and Logistics (CPSL-2). Ed.: D. Herberger, 622–633, publish-Ing. doi:10.15488/15270
Behrendt, S.; Wurster, M.; Strljic, M.; Klein, J.-F.; May, M. C.; Lanza, G.
2023. Proceedings of the 5th Conference on Production Systems and Logistics (CPSL-2). Ed.: D. Herberger, 622–633, publish-Ing. doi:10.15488/15270
Procedure Model for the Closed Loop Recycling of Electric and Electronic Devices; [Vorgehensmodell für die Kreislaufführung von Elektro- und Elektronikaltgeräten]
Wurster, M.; Kille, V.; Binding, M.; Lanza, G.
2023. ZWF Zeitschrift fuer Wirtschaftlichen Fabrikbetrieb, 118 (9), 599 – 604. doi:10.1515/zwf-2023-1117
Wurster, M.; Kille, V.; Binding, M.; Lanza, G.
2023. ZWF Zeitschrift fuer Wirtschaftlichen Fabrikbetrieb, 118 (9), 599 – 604. doi:10.1515/zwf-2023-1117
Extended Production Planning of Reconfigurable Manufacturing Systems by Means of Simulation-based Optimization
Behrendt, S.; Wurster, M.; May, M. C.; Lanza, G.
2023. 4th Conference on Production Systems and Logistics (CPSL 2023), 210–220, publish-Ing. doi:10.15488/13440
Behrendt, S.; Wurster, M.; May, M. C.; Lanza, G.
2023. 4th Conference on Production Systems and Logistics (CPSL 2023), 210–220, publish-Ing. doi:10.15488/13440
A Systematic Approach to Task Assignment and Production Planning in Disassembly with Employee Skills
Dvorak, J.; Wurster, M.; May, M. C.; Lanza, G.
2023. Procedia CIRP, 120, 958–963. doi:10.1016/j.procir.2023.09.107
Dvorak, J.; Wurster, M.; May, M. C.; Lanza, G.
2023. Procedia CIRP, 120, 958–963. doi:10.1016/j.procir.2023.09.107
An ontology for remanufacturing systems = Eine Ontologie für Remanufacturing-Systeme
Pfrommer, J.; Klein, J.-F.; Wurster, M.; Rapp, S.; Grauberger, P.; Lanza, G.; Albers, A.; Matthiesen, S.; Beyerer, J.
2022. at - Automatisierungstechnik, 70 (6), 534–541. doi:10.1515/auto-2021-0156
Pfrommer, J.; Klein, J.-F.; Wurster, M.; Rapp, S.; Grauberger, P.; Lanza, G.; Albers, A.; Matthiesen, S.; Beyerer, J.
2022. at - Automatisierungstechnik, 70 (6), 534–541. doi:10.1515/auto-2021-0156
Agiles Produktionssystem mittels lernender Roboter bei ungewissen Produktzuständen am Beispiel der Anlasser-Demontage = Concept of an agile production system based on learning robots applied to disassembly
Lanza, G.; Asfour, T.; Beyerer, J.; Deml, B.; Fleischer, J.; Heizmann, M.; Furmans, K.; Hofmann, C.; Cebulla, A.; Dreher, C.; Kaiser, J.-P.; Klein, J.-F.; Leven, F.; Mangold, S.; Mitschke, N.; Stricker, N.; Pfrommer, J.; Wu, C.; Wurster, M.; Zaremski, M.
2022. at - Automatisierungstechnik, 70 (6), 504–516. doi:10.1515/auto-2021-0158
Lanza, G.; Asfour, T.; Beyerer, J.; Deml, B.; Fleischer, J.; Heizmann, M.; Furmans, K.; Hofmann, C.; Cebulla, A.; Dreher, C.; Kaiser, J.-P.; Klein, J.-F.; Leven, F.; Mangold, S.; Mitschke, N.; Stricker, N.; Pfrommer, J.; Wu, C.; Wurster, M.; Zaremski, M.
2022. at - Automatisierungstechnik, 70 (6), 504–516. doi:10.1515/auto-2021-0158
Integrierte Steuerungsarchitektur für ein agiles Demontagesystem mit autonomer Produktbefundung = ntegrated control architecture for an agile disassembly system with autonomous product inspection
Wurster, M.; Klein, J.-F.; Kaiser, J.-P.; Mangold, S.; Furmans, K.; Heizmann, M.; Fleischer, J.; Lanza, G.
2022. at - Automatisierungstechnik, 70 (6), 542–556. doi:10.1515/auto-2021-0157
Wurster, M.; Klein, J.-F.; Kaiser, J.-P.; Mangold, S.; Furmans, K.; Heizmann, M.; Fleischer, J.; Lanza, G.
2022. at - Automatisierungstechnik, 70 (6), 542–556. doi:10.1515/auto-2021-0157
Potential assessment of an increased exchange of core information for remanufacturing in automotive reverse supply chains
Klenk, F.; Gallei, M.; Wurster, M.; Wagner, M.; Peukert, S.
2022. Procedia CIRP, 105, 446–451. doi:10.1016/j.procir.2022.02.074
Klenk, F.; Gallei, M.; Wurster, M.; Wagner, M.; Peukert, S.
2022. Procedia CIRP, 105, 446–451. doi:10.1016/j.procir.2022.02.074
Modelling and condition-based control of a flexible and hybrid disassembly system with manual and autonomous workstations using reinforcement learning
Wurster, M.; Michel, M.; May, M. C.; Kuhnle, A.; Stricker, N.; Lanza, G.
2022. Journal of Intelligent Manufacturing, 33 (2), 575–591. doi:10.1007/s10845-021-01863-3
Wurster, M.; Michel, M.; May, M. C.; Kuhnle, A.; Stricker, N.; Lanza, G.
2022. Journal of Intelligent Manufacturing, 33 (2), 575–591. doi:10.1007/s10845-021-01863-3
Industrie 4.0 im Remanufacturing : Analyse und Bewertung aktueller Forschungsansätze
Sprenger, K.; Klein, J.-F.; Wurster, M.; Stricker, N.; Lanza, G.; Furmans, K.
2021. Industrie 4.0 Management, 2021 (4), 37–40. doi:10.30844/I40M_21-4_S37-40
Sprenger, K.; Klein, J.-F.; Wurster, M.; Stricker, N.; Lanza, G.; Furmans, K.
2021. Industrie 4.0 Management, 2021 (4), 37–40. doi:10.30844/I40M_21-4_S37-40
Towards Ontology-based Autonomous Intralogistics for Agile Remanufacturing Production Systems
Klein, J.-F.; Wurster, M.; Stricker, N.; Lanza, G.; Furmans, K.
2021. Proceedings 2021 26th IEEE International Conference on Emerging Technologies and Factory Automation (ETFA): Västerås, Sweden – Online , 07-10 September, 2021, Institute of Electrical and Electronics Engineers (IEEE). doi:10.1109/ETFA45728.2021.9613486
Klein, J.-F.; Wurster, M.; Stricker, N.; Lanza, G.; Furmans, K.
2021. Proceedings 2021 26th IEEE International Conference on Emerging Technologies and Factory Automation (ETFA): Västerås, Sweden – Online , 07-10 September, 2021, Institute of Electrical and Electronics Engineers (IEEE). doi:10.1109/ETFA45728.2021.9613486
Towards planning and control in cognitive factories - A generic model including learning effects and knowledge transfer across system entities
Wurster, M.; Exner, Y.; Kaiser, J.-P.; Stricker, N.; Lanza, G.
2021. 9th CIRP Global Web Conference – Sustainable, resilient, and agile manufacturing and service operations : Lessons from COVID-19. Ed.: K. Medini, 158–163, Elsevier. doi:10.1016/j.procir.2021.10.025
Wurster, M.; Exner, Y.; Kaiser, J.-P.; Stricker, N.; Lanza, G.
2021. 9th CIRP Global Web Conference – Sustainable, resilient, and agile manufacturing and service operations : Lessons from COVID-19. Ed.: K. Medini, 158–163, Elsevier. doi:10.1016/j.procir.2021.10.025
Framework for simulation-based Trajectory Planning and Execution of Robots equipped with a Laser Scanner for Measurement and Inspection
Kaiser, J.-P.; Becker, S. N.; Wurster, M.; Stricker, N.; Lanza, G.
2021. 9th CIRP Global Web Conference – Sustainable, resilient, and agile manufacturing and service operations : Lessons from COVID-19. Ed.: K. Medini, 292–297, Elsevier. doi:10.1016/j.procir.2021.10.047
Kaiser, J.-P.; Becker, S. N.; Wurster, M.; Stricker, N.; Lanza, G.
2021. 9th CIRP Global Web Conference – Sustainable, resilient, and agile manufacturing and service operations : Lessons from COVID-19. Ed.: K. Medini, 292–297, Elsevier. doi:10.1016/j.procir.2021.10.047
Foresighted digital twin for situational agent selection in production control
May, M. C.; Overbeck, L.; Wurster, M.; Kuhnle, A.; Lanza, G.
2021. Procedia CIRP, 99, 27–32. doi:10.1016/j.procir.2021.03.005
May, M. C.; Overbeck, L.; Wurster, M.; Kuhnle, A.; Lanza, G.
2021. Procedia CIRP, 99, 27–32. doi:10.1016/j.procir.2021.03.005
Fluid Automation - A Definition and an Application in Remanufacturing Production Systems
Wurster, M.; Häfner, B.; Gauder, D.; Stricker, N.; Lanza, G.
2020. 8th CIRP Conference of Assembly Technology and Systems. Ed.: S. Makris, 508–513, Elsevier. doi:10.1016/j.procir.2020.05.267
Wurster, M.; Häfner, B.; Gauder, D.; Stricker, N.; Lanza, G.
2020. 8th CIRP Conference of Assembly Technology and Systems. Ed.: S. Makris, 508–513, Elsevier. doi:10.1016/j.procir.2020.05.267