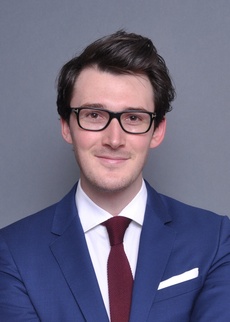
Louis Schäfer, M.Sc.
- Research Associate
- department: Production Systems
- office hours: to be agreed
- room: 013, Geb. 50.36
- phone: +49 1523 9502570
- louis schaefer ∂does-not-exist.kit edu
76131 Karlsruhe
Kaiserstraße 12
Louis Schäfer, M.Sc
Area of Research:
- Product-Production-Codesign
- Machine Learning in Production Planning and Control
- Industrie 4.0
General Tasks:
- Coordinator of the lecture ISS - Integrative Strategien und deren Umsetzung in Produktion und Entwicklung von Sportwagen
- Coordinator of the lecture SmartFactory∂Industry at Carl-Benz School of Engineering
- Coordinator of the lecture Teamproject Management and Technology
- Coordination Seminar “Process Mining in Production” with celonis and Bruker Switzerland
- Coordination Seminar "Data Mining in Production"
- Learning Factory on Lean Production and Industry 4.0
Projects:
- BMBF MoSyS - Human-oriented design of complex System of Systems
- BMBF teamIn - Digital leadership and technologies for the team interaction of tomorrow
- IFREE Grant H2R - Human & Robot Interaction
Curriculum Vitae:
since 10/2020 |
Research Associate at the Institute of Production Science (wbk) at Karlsruhe Institute of Technology (KIT) |
10/2014-08/2020 |
Study of Mechanical Engineering at Karlsruhe Institute of Technology (KIT) |
Publications
A systematic approach for simulation-based dimensioning of production systems during the concept phase of factory planning
Schäfer, L.; Klenk, F.; Maier, T.; Zehner, M.; Peukert, S.; Linzbach, R.; Treiber, T.; Lanza, G.
2024. Production Engineering. doi:10.1007/s11740-024-01273-3
Schäfer, L.; Klenk, F.; Maier, T.; Zehner, M.; Peukert, S.; Linzbach, R.; Treiber, T.; Lanza, G.
2024. Production Engineering. doi:10.1007/s11740-024-01273-3
Reinforcement learning for energy-efficient control of parallel and identical machines
Loffredo, A.; May, M. C.; Schäfer, L.; Matta, A.; Lanza, G.
2023. CIRP Journal of Manufacturing Science and Technology, 44, 91–103. doi:10.1016/j.cirpj.2023.05.007
Loffredo, A.; May, M. C.; Schäfer, L.; Matta, A.; Lanza, G.
2023. CIRP Journal of Manufacturing Science and Technology, 44, 91–103. doi:10.1016/j.cirpj.2023.05.007
Reinforcement Learning for Improvement Measure Selection in Learning Factories
May, M. C.; Hermeler, S.; Mauch, E.; Dvorak, J.; Schäfer, L.; Lanza, G.
2023. Proceedings of the 13th Conference on Learning Factories (CLF 2023). Hrsg.: Hummel, Vera, Social Science Electronic Publishing. doi:10.2139/ssrn.4470426
May, M. C.; Hermeler, S.; Mauch, E.; Dvorak, J.; Schäfer, L.; Lanza, G.
2023. Proceedings of the 13th Conference on Learning Factories (CLF 2023). Hrsg.: Hummel, Vera, Social Science Electronic Publishing. doi:10.2139/ssrn.4470426
Planning and Multi-Objective Optimization of Production Systems by means of Assembly Line Balancing
Schäfer, L.; Kochendörfer, P.; May, M. C.; Lanza, G.
2023. Procedia CIRP, 120, 1125 – 1130. doi:10.1016/j.procir.2023.09.136
Schäfer, L.; Kochendörfer, P.; May, M. C.; Lanza, G.
2023. Procedia CIRP, 120, 1125 – 1130. doi:10.1016/j.procir.2023.09.136
Prosocial behavior among human workers in robot-augmented production teams : a field-in-the-lab experiment
Gorny, P. M.; Renner, B.; Schäfer, L.
2023. Frontiers in Behavioral Economics, 2. doi:10.3389/frbhe.2023.1220563
Gorny, P. M.; Renner, B.; Schäfer, L.
2023. Frontiers in Behavioral Economics, 2. doi:10.3389/frbhe.2023.1220563
Systematics for an Integrative Modelling of Product and Production System
Schäfer, L.; Günther, M.; Martin, A.; Lüpfert, M.; Mandel, C.; Rapp, S.; Lanza, G.; Anacker, H.; Albers, A.; Köchling, D.
2023. 16th CIRP Conference on Intelligent Computation in Manufacturing Engineering (CIRP ICME 2023), 118, 104–109. doi:10.1016/j.procir.2023.06.019
Schäfer, L.; Günther, M.; Martin, A.; Lüpfert, M.; Mandel, C.; Rapp, S.; Lanza, G.; Anacker, H.; Albers, A.; Köchling, D.
2023. 16th CIRP Conference on Intelligent Computation in Manufacturing Engineering (CIRP ICME 2023), 118, 104–109. doi:10.1016/j.procir.2023.06.019
Towards Product-Production-CoDesign for the Production of the Future
May, M. C.; Schäfer, L.; Frey, A.; Krahe, C.; Lanza, G.
2023. Procedia CIRP, 119, 944–949. doi:10.1016/j.procir.2023.02.172
May, M. C.; Schäfer, L.; Frey, A.; Krahe, C.; Lanza, G.
2023. Procedia CIRP, 119, 944–949. doi:10.1016/j.procir.2023.02.172
Classifying Parts using Feature Extraction and Similarity Assessment
Schäfer, L.; Treml, N.; May, M. C.; Lanza, G.
2023. Procedia CIRP, 119, 822–827. doi:10.1016/j.procir.2023.03.127
Schäfer, L.; Treml, N.; May, M. C.; Lanza, G.
2023. Procedia CIRP, 119, 822–827. doi:10.1016/j.procir.2023.03.127
Explainable reinforcement learning in production control of job shop manufacturing system
Kuhnle, A.; May, M. C.; Schäfer, L.; Lanza, G.
2022. International Journal of Production Research, 60 (19), 5812–5834. doi:10.1080/00207543.2021.1972179
Kuhnle, A.; May, M. C.; Schäfer, L.; Lanza, G.
2022. International Journal of Production Research, 60 (19), 5812–5834. doi:10.1080/00207543.2021.1972179
Produkt-Produktions-CoDesign: Ein Ansatz zur integrierten Produkt- und Produktionssystementwickung über Generationen und Lebenszyklen hinweg
Albers, A.; Rapp, S.; Klippert, M.; Lanza, G.; Schäfer, L.
2022. News / Wissenschaftliche Gesellschaft für Produktentwicklung, WiGeP, Berliner Kreis & WGMK, (1), 3
Albers, A.; Rapp, S.; Klippert, M.; Lanza, G.; Schäfer, L.
2022. News / Wissenschaftliche Gesellschaft für Produktentwicklung, WiGeP, Berliner Kreis & WGMK, (1), 3
New Competences in a Digitalized Shopfloor – A Modular Training Concept for Learning Factories
Schäfer, L.; Ströhlein, K.; Kandler, M.; Hulla, M.; Ast, J.; Lanza, G.; Nieken, P.; Ramsauer, C.; Nyhuis, P.
2022. SSRN eLibrary, Art.-Nr. 4071822. doi:10.2139/ssrn.4071822
Schäfer, L.; Ströhlein, K.; Kandler, M.; Hulla, M.; Ast, J.; Lanza, G.; Nieken, P.; Ramsauer, C.; Nyhuis, P.
2022. SSRN eLibrary, Art.-Nr. 4071822. doi:10.2139/ssrn.4071822
Decision Experiments in the Learning Factory: A Proof of Concept
Ströhlein, K.; Gorny, P. M.; Kandler, M.; Schäfer, L.; Nieken, P.; Lanza, G.
2022. Proceedings of the 12th Conference on Learning Factories (CLF 2022), Art.-Nr. 4072356, SSRN. doi:10.2139/ssrn.4072356
Ströhlein, K.; Gorny, P. M.; Kandler, M.; Schäfer, L.; Nieken, P.; Lanza, G.
2022. Proceedings of the 12th Conference on Learning Factories (CLF 2022), Art.-Nr. 4072356, SSRN. doi:10.2139/ssrn.4072356
Applying Natural Language Processing in Manufacturing
May, M. C.; Neidhöfer, J.; Körner, T.; Schäfer, L.; Lanza, G.
2022. Procedia CIRP, 10th CIRP Global Web Conference – Material Aspects of Manufacturing Processes, 115, 184–189. doi:10.1016/j.procir.2022.10.071
May, M. C.; Neidhöfer, J.; Körner, T.; Schäfer, L.; Lanza, G.
2022. Procedia CIRP, 10th CIRP Global Web Conference – Material Aspects of Manufacturing Processes, 115, 184–189. doi:10.1016/j.procir.2022.10.071
Shopfloor Management Acceptance in Global Manufacturing
Kandler, M.; Dierolf, L.; Bender, M.; Schäfer, L.; May, M. C.; Lanza, G.
2022. Procedia CIRP, 10th CIRP Global Web Conference – Material Aspects of Manufacturing Processes, 115, 190–195. doi:10.1016/j.procir.2022.10.072
Kandler, M.; Dierolf, L.; Bender, M.; Schäfer, L.; May, M. C.; Lanza, G.
2022. Procedia CIRP, 10th CIRP Global Web Conference – Material Aspects of Manufacturing Processes, 115, 190–195. doi:10.1016/j.procir.2022.10.072
Lösungsmuster zur Produktionssystemplanung : Entwicklung eines integrierten Rahmenmodells und exemplarische Anwendung = Solution Patterns for Production System Planning – Development of an Integrated Framework Model and Exemplary Application
Schäfer, L.; Reichardt, A.-K.; Lanza, G.
2022. wt Werkstattstechnik online, 112 (04), 243–247. doi:10.37544/1436-4980-2022-04-43
Schäfer, L.; Reichardt, A.-K.; Lanza, G.
2022. wt Werkstattstechnik online, 112 (04), 243–247. doi:10.37544/1436-4980-2022-04-43
Digitale Transformation in global produzierenden Unternehmen = Digital Transformation in global manufacturing companies
Steier, G.; Schäfer, L.; Moser, S.; Kandler, M.; Lanza, G.
2022. WT Werkstattstechnik, 112 (5), 314–319. doi:10.37544/1436–4980–2022–5–44
Steier, G.; Schäfer, L.; Moser, S.; Kandler, M.; Lanza, G.
2022. WT Werkstattstechnik, 112 (5), 314–319. doi:10.37544/1436–4980–2022–5–44
KI-Assistenzsysteme in der Produktentwicklung
Schäfer, L.; Krahe, C.
2022. Potentiale digitaler Führung und Technologien für die Teaminteraktion von morgen : Zwischenbericht des vom BMBF geförderten Forschungs- und Entwicklungsprojektes im Rahmen des Programms. Hrsg.: G. Lanza, 68 – 74, TEWISS Verl
Schäfer, L.; Krahe, C.
2022. Potentiale digitaler Führung und Technologien für die Teaminteraktion von morgen : Zwischenbericht des vom BMBF geförderten Forschungs- und Entwicklungsprojektes im Rahmen des Programms. Hrsg.: G. Lanza, 68 – 74, TEWISS Verl
Automated Derivation of Optimal Production Sequences from Product Data
Schäfer, L.; Frank, A.; May, M. C.; Lanza, G.
2022. Procedia CIRP, 107, 469–474. doi:10.1016/j.procir.2022.05.010
Schäfer, L.; Frank, A.; May, M. C.; Lanza, G.
2022. Procedia CIRP, 107, 469–474. doi:10.1016/j.procir.2022.05.010
Product-Production-CoDesign: An Approach on Integrated Product and Production Engineering Across Generations and Life Cycles
Albers, A.; Lanza, G.; Klippert, M.; Schäfer, L.; Frey, A.; Hellweg, F.; Müller-Welt, P.; Schöck, M.; Krahe, C.; Nowoseltschenko, K.; Rapp, S.
2022. 32nd CIRP Design Conference (CIRP Design 2022) - Design in a changing world. Ed.: N. Anwer, 167–172, Elsevier. doi:10.1016/j.procir.2022.05.231
Albers, A.; Lanza, G.; Klippert, M.; Schäfer, L.; Frey, A.; Hellweg, F.; Müller-Welt, P.; Schöck, M.; Krahe, C.; Nowoseltschenko, K.; Rapp, S.
2022. 32nd CIRP Design Conference (CIRP Design 2022) - Design in a changing world. Ed.: N. Anwer, 167–172, Elsevier. doi:10.1016/j.procir.2022.05.231
Learning Factory Labs as Field-in-the-Lab Environments – An Experimental Concept for Human-Centred Production Research
Kandler, M.; Schäfer, L.; Gorny, P. M.; Ströhlein, K.; Lanza, G.; Nieken, P.
2021. Proceedings of the 11th Conference on Learning Factories (CLF)
Kandler, M.; Schäfer, L.; Gorny, P. M.; Ströhlein, K.; Lanza, G.; Nieken, P.
2021. Proceedings of the 11th Conference on Learning Factories (CLF)
Towards a User Support System for Computed Tomography Measurements Using Machine Learning
Höger, K.; Schäfer, L.; Schild, L.; Lanza, G.
2021. Production at the Leading Edge of Technology: Proceedings of the 11th Congress of the German Academic Association for Production Technology (WGP), Dresden, September 2021. Edited by Bernd-Arno Behrens, Alexander Brosius, Welf-Guntram Drossel, Wolfgang Hintze, Steffen Ihlenfeldt, Peter Nyhuis, 506–514, Springer. doi:10.1007/978-3-030-78424-9_56
Höger, K.; Schäfer, L.; Schild, L.; Lanza, G.
2021. Production at the Leading Edge of Technology: Proceedings of the 11th Congress of the German Academic Association for Production Technology (WGP), Dresden, September 2021. Edited by Bernd-Arno Behrens, Alexander Brosius, Welf-Guntram Drossel, Wolfgang Hintze, Steffen Ihlenfeldt, Peter Nyhuis, 506–514, Springer. doi:10.1007/978-3-030-78424-9_56
Queue Length Forecasting in Complex Manufacturing Job Shops
May, M. C.; Albers, A.; Fischer, M. D.; Mayerhofer, F.; Schäfer, L.; Lanza, G.
2021. Forecasting, 2021 (2), 322–338. doi:10.3390/forecast3020021
May, M. C.; Albers, A.; Fischer, M. D.; Mayerhofer, F.; Schäfer, L.; Lanza, G.
2021. Forecasting, 2021 (2), 322–338. doi:10.3390/forecast3020021
Integriertes Produkt-Produktions-Codesign : Ganzheitliche Auswirkungsanalysen von Änderungsfällen und Identifikation von Lösungsmustern = Integrated product-production codesign
Schäfer, L.; Burkhardt, L.; Kuhnle, A.; Lanza, G.
2021. wt Werkstattstechnik online, 111 (4), 201–205. doi:10.37544/1436-4980-2021-04-23
Schäfer, L.; Burkhardt, L.; Kuhnle, A.; Lanza, G.
2021. wt Werkstattstechnik online, 111 (4), 201–205. doi:10.37544/1436-4980-2021-04-23
Design, Implementation and Evaluation of Reinforcement Learning for an Adaptive Order Dispatching in Job Shop Manufacturing Systems
Kuhnle, A.; Schäfer, L.; Stricker, N.; Lanza, G.
2019. Procedia CIRP, 81, 234–239. doi:10.1016/j.procir.2019.03.041
Kuhnle, A.; Schäfer, L.; Stricker, N.; Lanza, G.
2019. Procedia CIRP, 81, 234–239. doi:10.1016/j.procir.2019.03.041