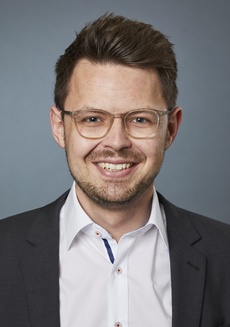
Jan-Philipp Kaiser, M.Sc.
- Research Associate
- department: Production Systems
- office hours: to be agreed
- room: 111, Geb. 50.36
- phone: +49 1523 9502650
- Jan-Philipp Kaiser ∂does-not-exist.kit edu
76131 Karlsruhe
Kaiserstraße 12
Jan-Philipp Kaiser, M.Sc.
Area of Research:
- Circular Production
- Robotics
- Artificial Intelligence
General Tasks:
Projects:
- AgiProbot
- DemoBat
- Reflexbat
- FsReman
Curriculum Vitae:
since 11/2019 |
Research Associate at the Institute of Production Science (wbk) at Karlsruhe Institute of Technology (KIT) |
10/2012-10/2019 |
Study of Mechanical engineering at Karlsruhe Institute of Technology (KIT) |
|
Publications
6D Pose Estimation on Point Cloud Data through Prior Knowledge Integration: A Case Study in Autonomous Disassembly
Wu, C.; Fu, H.; Kaiser, J.-P.; Barczak, E. T.; Pfrommer, J.; Lanza, G.; Heizmann, M.; Beyerer, J.
2024. Procedia CIRP, 122, 193 – 198. doi:10.1016/j.procir.2024.01.028
Wu, C.; Fu, H.; Kaiser, J.-P.; Barczak, E. T.; Pfrommer, J.; Lanza, G.; Heizmann, M.; Beyerer, J.
2024. Procedia CIRP, 122, 193 – 198. doi:10.1016/j.procir.2024.01.028
Anomaly Detection in Li-ion cell Contacting – Innovative Anomaly Detection in Laser Welding: A Pipeline Based on Radiation Emission Analysis and Machine Learning.; [Anomalieerkennung bei der Li-Ionen-Zellkontaktierung]
Merz, D.; Gerlitz, E.; Kaiser, J.-P.; Fleischer, J.
2023. ZWF Zeitschrift fuer Wirtschaftlichen Fabrikbetrieb, 118 (11), 790 – 794. doi:10.1515/zwf-2023-1146
Merz, D.; Gerlitz, E.; Kaiser, J.-P.; Fleischer, J.
2023. ZWF Zeitschrift fuer Wirtschaftlichen Fabrikbetrieb, 118 (11), 790 – 794. doi:10.1515/zwf-2023-1146
Assessing the optical configuration of a structured light scanner in metrological use
Schild, L.; Sasse, F.; Kaiser, J.-P.; Lanza, G.
2022. Measurement Science and Technology, 33 (8), Art.-Nr.: 085018. doi:10.1088/1361-6501/ac6e2f
Schild, L.; Sasse, F.; Kaiser, J.-P.; Lanza, G.
2022. Measurement Science and Technology, 33 (8), Art.-Nr.: 085018. doi:10.1088/1361-6501/ac6e2f
Agiles Produktionssystem mittels lernender Roboter bei ungewissen Produktzuständen am Beispiel der Anlasser-Demontage = Concept of an agile production system based on learning robots applied to disassembly
Lanza, G.; Asfour, T.; Beyerer, J.; Deml, B.; Fleischer, J.; Heizmann, M.; Furmans, K.; Hofmann, C.; Cebulla, A.; Dreher, C.; Kaiser, J.-P.; Klein, J.-F.; Leven, F.; Mangold, S.; Mitschke, N.; Stricker, N.; Pfrommer, J.; Wu, C.; Wurster, M.; Zaremski, M.
2022. at - Automatisierungstechnik, 70 (6), 504–516. doi:10.1515/auto-2021-0158
Lanza, G.; Asfour, T.; Beyerer, J.; Deml, B.; Fleischer, J.; Heizmann, M.; Furmans, K.; Hofmann, C.; Cebulla, A.; Dreher, C.; Kaiser, J.-P.; Klein, J.-F.; Leven, F.; Mangold, S.; Mitschke, N.; Stricker, N.; Pfrommer, J.; Wu, C.; Wurster, M.; Zaremski, M.
2022. at - Automatisierungstechnik, 70 (6), 504–516. doi:10.1515/auto-2021-0158
Integrierte Steuerungsarchitektur für ein agiles Demontagesystem mit autonomer Produktbefundung = ntegrated control architecture for an agile disassembly system with autonomous product inspection
Wurster, M.; Klein, J.-F.; Kaiser, J.-P.; Mangold, S.; Furmans, K.; Heizmann, M.; Fleischer, J.; Lanza, G.
2022. at - Automatisierungstechnik, 70 (6), 542–556. doi:10.1515/auto-2021-0157
Wurster, M.; Klein, J.-F.; Kaiser, J.-P.; Mangold, S.; Furmans, K.; Heizmann, M.; Fleischer, J.; Lanza, G.
2022. at - Automatisierungstechnik, 70 (6), 542–556. doi:10.1515/auto-2021-0157
Systematic Identification of Hazardous States and Approach for Condition Monitoring in the Context of Li-ion Battery Disassembly
Gerlitz, E.; Greifenstein, M.; Kaiser, J.-P.; Mayer, D.; Lanza, G.; Fleischer, J.
2022. Procedia CIRP, 107, 308–313. doi:10.1016/j.procir.2022.04.050
Gerlitz, E.; Greifenstein, M.; Kaiser, J.-P.; Mayer, D.; Lanza, G.; Fleischer, J.
2022. Procedia CIRP, 107, 308–313. doi:10.1016/j.procir.2022.04.050
MotorFactory: A Blender Add-on for Large Dataset Generation of Small Electric Motors
Wu, C.; Zhou, K.; Kaiser, J.-P.; Mitschke, N.; Klein, J.-F.; Pfrommer, J.; Beyerer, J.; Lanza, G.; Heizmann, M.; Furmans, K.
2022. Procedia CIRP, 106, 138–143. doi:10.1016/j.procir.2022.02.168
Wu, C.; Zhou, K.; Kaiser, J.-P.; Mitschke, N.; Klein, J.-F.; Pfrommer, J.; Beyerer, J.; Lanza, G.; Heizmann, M.; Furmans, K.
2022. Procedia CIRP, 106, 138–143. doi:10.1016/j.procir.2022.02.168
Konzept einer automatisierten und modularen Befundungsstation in der wandlungsfähigen Produktion – Am Anwendungsfall des Remanufacturings = Concept of an Automated and Modular Diagnostic Station in Adaptable Production Based on the Application of Remanufacturing
Kaiser, J.-P.; Mitschke, N.; Stricker, N.; Heizmann, M.; Lanza, G.
2021. Zeitschrift für wirtschaftlichen Fabrikbetrieb, 116 (5), 313–317. doi:10.1515/zwf-2021-0070
Kaiser, J.-P.; Mitschke, N.; Stricker, N.; Heizmann, M.; Lanza, G.
2021. Zeitschrift für wirtschaftlichen Fabrikbetrieb, 116 (5), 313–317. doi:10.1515/zwf-2021-0070
View-Planning im Remanufacturing : Optisches Erfassen von Produkten im Remanufacturing durch View-Planning = Optical acquisition of products in remanufacturing by view-planning – View-Planning in Remanufacturing
Kaiser, J.-P.; Bolender, M.; Eschner, N.; Lanza, G.
2021. WT Werkstattstechnik, 111 (11-12), 781–785. doi:10.37544/1436-4980-2021-11-12-11
Kaiser, J.-P.; Bolender, M.; Eschner, N.; Lanza, G.
2021. WT Werkstattstechnik, 111 (11-12), 781–785. doi:10.37544/1436-4980-2021-11-12-11
Towards planning and control in cognitive factories - A generic model including learning effects and knowledge transfer across system entities
Wurster, M.; Exner, Y.; Kaiser, J.-P.; Stricker, N.; Lanza, G.
2021. 9th CIRP Global Web Conference – Sustainable, resilient, and agile manufacturing and service operations : Lessons from COVID-19. Ed.: K. Medini, 158–163, Elsevier. doi:10.1016/j.procir.2021.10.025
Wurster, M.; Exner, Y.; Kaiser, J.-P.; Stricker, N.; Lanza, G.
2021. 9th CIRP Global Web Conference – Sustainable, resilient, and agile manufacturing and service operations : Lessons from COVID-19. Ed.: K. Medini, 158–163, Elsevier. doi:10.1016/j.procir.2021.10.025
Framework for simulation-based Trajectory Planning and Execution of Robots equipped with a Laser Scanner for Measurement and Inspection
Kaiser, J.-P.; Becker, S. N.; Wurster, M.; Stricker, N.; Lanza, G.
2021. 9th CIRP Global Web Conference – Sustainable, resilient, and agile manufacturing and service operations : Lessons from COVID-19. Ed.: K. Medini, 292–297, Elsevier. doi:10.1016/j.procir.2021.10.047
Kaiser, J.-P.; Becker, S. N.; Wurster, M.; Stricker, N.; Lanza, G.
2021. 9th CIRP Global Web Conference – Sustainable, resilient, and agile manufacturing and service operations : Lessons from COVID-19. Ed.: K. Medini, 292–297, Elsevier. doi:10.1016/j.procir.2021.10.047
Designing an adaptive production control system using reinforcement learning
Kuhnle, A.; Kaiser, J.-P.; Theiß, F.; Stricker, N.; Lanza, G.
2021. Journal of intelligent manufacturing, 32, 855–876. doi:10.1007/s10845-020-01612-y
Kuhnle, A.; Kaiser, J.-P.; Theiß, F.; Stricker, N.; Lanza, G.
2021. Journal of intelligent manufacturing, 32, 855–876. doi:10.1007/s10845-020-01612-y