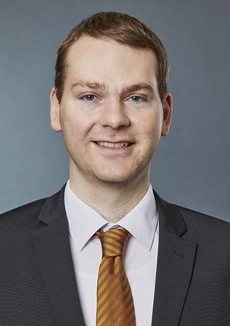
Dr.-Ing. Marvin May
- PostDoc Production Systems
- department: Production Systems
- office hours: to be agreed
- room: 110, Geb. 50.36
- phone: +49 1523 9502624
- fax: +49 721 608 - 45005
- Marvin May ∂does-not-exist.kit edu
76131 Karlsruhe
Kaiserstraße 12Links:
Dr.-Ing. Marvin May
Area of Research:
-
Machine Learning and AI in Manufacturing
-
Semiconductor Manufacturing
-
Production Control of flexible Production systems
-
Intelligent Production Planning
-
Digital Twin, Industrial Internet of Things and Simulations
-
Software-Defined Manufacturing and resilient Production
-
Knowledge Engineering and Large Language Models (LLMs)
-
Design and Management of Circular Production
-
Product-Production-CoDesign
-
Industrie 4.0 and Digitalization strategy in Production Networks
General Tasks:
- PostDoc Production Systems
- CIRP Research Affiliate
- Coordination of Research focus area Industrie 4.0
- Lectures
- Operations Management
- Data Mining in Production and Process Mining in Production (Seminars)
- Integrated production planning and Control in the age of Industry 4.0 (IPP)
- SmartFactory@Industry
- Learning factory on global production
Dissertation:
Intelligent production control for time-constrained complex job shops - doi:10.5445/IR/1000169066
Projects:
- BMWi SDM4FZI – Software-Defined Manufacturing für die Fahrzeug- und Zulieferindustrie
-
EU MCSA Digiman4.0 - DIGItal MANufacturing Technologies for Zero-defect Industry 4.0 Production is a world excellent research training to 15 ESRs
-
Innovation Center SAP – Development of an AI-based multi-agent production control for matrix production
-
BaWü Robust - Assistance system for rule-based robustness enhancement of interlinked production systems
-
DFG SFB 1574 - Circular Factory
-
DFG SPP 2187 - Tolerance-free series production of high-performance concrete components through transient-interactive coupling of design and production
-
BMWK CliCE-DiPP – Climate-neutral economy enabled by Digital Product Carbon Passport
-
ICM SDSeq - Software-Defined Model and Function Sequencing for Integrated Launching of Modular Production Systems
-
ICM GAIA-X4ICM - Infrastructure for end-to-end digitalization of production based on Gaia-X
-
BMBF KARL - Competence Center KARL: Artificial intelligence for work and learning in the Karlsruhe region
-
BMBF ChampI4.0ns - Intelligent and sovereign use of data using the timber industry as an example
-
Innovation Center Bosch – Agile Production System
-
AgiloBat - Agile battery cell production
-
DiKliMa - Digitalization, climate management & employee recruitment and retention
-
BMBF MoSyS - Human-centered design of complex systems of systems
-
BMBF teamIn - Digital leadership and technologies for the team interaction of tomorrow
-
EU Digiprime – Digital Platform for Circular Economy
Curriculum Vitae:
07/2024 |
Dissertation Award: Dr.-Ing. Willy Höfler prize of the department of mechanical Engineering (KIT) |
2024 |
BMBF Exist academic supervisor puerro |
2020-2024 |
Academic supervisor enactus e.V. Karlsruhe |
since 2024 |
PostDoc |
since 09/2021 |
Chief Engineer / Team lead of the team “Production System Planning” |
09/2022-11/2022 | Visiting Researcher National University of Singapore (NUS) |
09/2019-08/2021 | Research Associate at the Institute of Production Science (wbk) at Karlsruhe Institute of Technology (KIT) |
10/2016-08/2019 |
Industrial Engineering and Management (M.Sc.) as well as Information Systems (M.Sc.) at Karlsruhe Institute of Technology (KIT) |
02/2019-07/2019 | International Business at Shanghai Jiaotong University (上海交通大学) |
09/2017-09/2018 |
Mechanical & Industrial Engineering and Graduate Teaching Assistant in Resource Economics at the University of Massachusetts, Amherst (UMass) |
10/2013-09/2016 | Industrial Engineering and Management (B.Sc.) at Karlsruhe Institute of Technology (KIT) |
Publications
Schäfer, L.; Tse, S.; May, M. C.; Lanza, G.
2025. Journal of Manufacturing Systems, 78, 109 – 123. doi:10.1016/j.jmsy.2024.11.008
Hermann, Y.; Patlakis, C.; Hörger, M.; May, M. C.; Lanza, G.
2025. Procedia CIRP, 132, 221 – 226. doi:10.1016/j.procir.2025.01.037
Rechkemmer, D.; Korth, M.; May, M. C.; Lanza, G.
2025. Procedia CIRP, 132, 110–115. doi:10.1016/j.procir.2025.01.019
May, M. C.; Schäfer, L.; Lachnit, T.; Lanza, G.
2025. Sustainable Manufacturing as a Driver for Growth – Proceedings of the 19th Global Conference on Sustainable Manufacturing, December 4–6, 2023, Buenos Aires, Argentina. Ed.: H. Kohl, 185–193, Springer Nature Switzerland. doi:10.1007/978-3-031-77429-4_21
Behrendt, S.; Altenmüller, T.; May, M. C.; Kuhnle, A.; Lanza, G.
2025. Journal of Intelligent Manufacturing. doi:10.1007/s10845-025-02572-x
May, M. C.; Kiefer, L.; Lanza, G.
2024. 2024 Winter Simulation Conference (WSC), 1943 – 1954, Institute of Electrical and Electronics Engineers (IEEE). doi:10.1109/WSC63780.2024.10838845
Kim, Y. H.; Ye, W.; Kumar, R.; Bail, F.; Dvorak, J.; Tan, Y.; May, M. C.; Chang, Q.; Athinarayanan, R.; Lanza, G.; Sutherland, J. W.; Li, X.; Nath, C.
2024. Algorithms, 17 (562), n.a. doi:10.3390/a17120562
May, M. C.; Oberst, J.; Lanza, G.
2024. Journal of Intelligent Manufacturing, 35 (8), 4259–4276. doi:10.1007/s10845-024-02472-6
Loffredo, A.; May, M. C.; Matta, A.; Lanza, G.
2024. Journal of Intelligent Manufacturing, 35 (8), 3775–3791. doi:10.1007/s10845-023-02258-2
Gartner, P.; Bilger, M.; Wurster, M.; Kandler, M.; May, M.; Lanza, G.
2024. Procedia CIRP, 130, 1256 – 1261. doi:10.1016/j.procir.2024.10.236
Ye, W.; Dvorak, J.; Li, X.; May, M. C.
2024. 57th CIRP Conference on Manufacturing Systems 2024 Hrsg.: Putnik, Goran, 130, 1695–1700. doi:10.1016/j.procir.2024.10.302
Alt, B.; Zahn, J.; Kienle, C.; Dvorak, J.; May, M.; Katic, D.; Jäkel, R.; Kopp, T.; Beetz, M.; Lanza, G.
2024. Procedia CIRP, 130, 591–596. doi:10.1016/j.procir.2024.10.134
Kaiser, J.-P.; Koch, D.; Gäbele, J.; May, M. C.; Lanza, G.
2024. CIRP Journal of Manufacturing Science and Technology, 53, 128–138. doi:10.1016/j.cirpj.2024.07.006
Fleischer, J.; Zanger, F.; Schulze, V.; Neumann, G.; Stricker, N.; Furmans, K.; Pfrommer, J.; Lanza, G.; Hansjosten, M.; Fischmann, P.; Dvorak, J.; Klein, J.-F.; Rauscher, F.; Ebner, A.; May, M. C.; Gönnheimer, P.
2024. at - Automatisierungstechnik, 72 (9), 861–874. doi:10.1515/auto-2024-0005
Dvorak, J.; Hörsting, R.; Gleich, K.; Litterst, J.; May, M. C.; Lanza, G.
2024. Learning Factories of the Future ; Proceedings of the 14th Conference on Learning Factories 2024, Volume 1, Ed.: S. Thiede, 358 – 365, Springer Nature Switzerland. doi:10.1007/978-3-031-65411-4_42
Schäfer, L.; Tse, S.; May, M. C.; Lanza, G.
2024. EurOMA - Transforming People and Processes for a better World, Barcelona, 29.06.-04.07.2024
Dvorak, J.; Amma, C.; Kandler, M.; Clever, F.; May, M. C.; Lanza, G.
2024. ZWF Zeitschrift fuer Wirtschaftlichen Fabrikbetrieb, 119 (6), 470 – 473. doi:10.1515/zwf-2024-1080
May, M. C.
2024, March 27. Shaker Verlag. doi:10.5445/IR/1000169066
May, M. C.; Glatter, D.; Arnold, D.; Pfeffer, D.; Lanza, G.
2024. Journal of Manufacturing Systems, 72, 437 – 459. doi:10.1016/j.jmsy.2023.12.001
May, M. C.; Nestroy, C.; Overbeck, L.; Lanza, G.
2024. International Journal of Production Research, 62 (1-2), 141–156. doi:10.1080/00207543.2023.2284833
May, M. C.; Finke, A.; Theuner, K.; Lanza, G.
2024. Procedia CIRP, 121, 49 – 54. doi:10.1016/j.procir.2023.11.002
Tabar, R. S.; Magnanini, M. C.; Stamer, F.; May, M. C.; Lanza, G.; Wärmefjord, K.; Söderberg, R.
2024. Procedia CIRP, 121, 1 – 6. doi:10.1016/j.procir.2023.09.221
Behrendt, S.; Stamer, F.; May, M. C.; Lanza, G.
2023. Proceedings of the 5th Conference on Production Systems and Logistics (CPSL-2). Ed.: D. Herberger, 255–267, publish-Ing. doi:10.15488/15271
Behrendt, S.; Wurster, M.; Strljic, M.; Klein, J.-F.; May, M. C.; Lanza, G.
2023. Proceedings of the 5th Conference on Production Systems and Logistics (CPSL-2). Ed.: D. Herberger, 622–633, publish-Ing. doi:10.15488/15270
Loffredo, A.; May, M. C.; Matta, A.
2023. Italian Manufacturing Association Conference, 428 – 436, Materials Research Forum LLC. doi:10.21741/9781644902714-51
Behrendt, S.; Ungen, M.; Fisel, J.; Hung, K.-C.; May, M.-C.; Leberle, U.; Lanza, G.
2023. A. B. T. M. H.-C. Liewald Mathiasand Verl (Ed.), Production at the Leading Edge of Technology – Proceedings of the 12th Congress of the German Academic Association for Production Technology (WGP), University of Stuttgart, October 2022. Ed.: M. Liewald, 705–716, Springer International Publishing. doi:10.1007/978-3-031-18318-8_70
Loffredo, A.; May, M. C.; Schäfer, L.; Matta, A.; Lanza, G.
2023. CIRP Journal of Manufacturing Science and Technology, 44, 91–103. doi:10.1016/j.cirpj.2023.05.007
Overbeck, L.; Glaser, V.; May, M. C.; Lanza, G.
2023. Production Processes and Product Evolution in the Age of Disruption. Ed.: F. Galizia, 338–346, Springer International Publishing. doi:10.1007/978-3-031-34821-1_37
May, M. C.; Hermeler, S.; Mauch, E.; Dvorak, J.; Schäfer, L.; Lanza, G.
2023. Proceedings of the 13th Conference on Learning Factories (CLF 2023). Hrsg.: Hummel, Vera, Social Science Electronic Publishing. doi:10.2139/ssrn.4470426
Bilen, A.; Stamer, F.; May, M. C.; Lanza, G.
2023. Conference Proceedings : 23rd International Conference & Exhibition, 1–4, Euspen
Behrendt, S.; Martin, M.; Puchta, A.; Ströbel, R.; Fisel, J.; May, M.; Gönnheimer, P.; Fleischer, J.; Lanza, G.
2023. Advances in Automotive Production Technology – Towards Software-Defined Manufacturing and Resilient Supply Chains : Stuttgart Conference on Automotive Production (SCAP2022). Ed.: N. Kiefl, 25–34, Springer International Publishing. doi:10.1007/978-3-031-27933-1_3
Behrendt, S.; Wurster, M.; May, M. C.; Lanza, G.
2023. 4th Conference on Production Systems and Logistics (CPSL 2023), 210–220, publish-Ing. doi:10.15488/13440
Chen, T.; Sampath, V.; May, M. C.; Shan, S.; Jorg, O. J.; Aguilar Martín, J. J.; Stamer, F.; Fantoni, G.; Tosello, G.; Calaon, M.
2023. Applied Sciences (Switzerland), 13 (3), Art.-Nr.: 1903. doi:10.3390/app13031903
Dvorak, J.; Wurster, M.; May, M. C.; Lanza, G.
2023. Procedia CIRP, 120, 958–963. doi:10.1016/j.procir.2023.09.107
May, M. C.; Kiefer, L.; Frey, A.; Duffie, N. A.; Lanza, G.
2023. Procedia CIRP, 120, 147 – 152. doi:10.1016/j.procir.2023.08.027
Kandler, M.; Seibert, C.; May, M. C.; Lanza, G.
2023. Procedia CIRP, 120, 1321 – 1326. doi:10.1016/j.procir.2023.09.170
Schäfer, L.; Kochendörfer, P.; May, M. C.; Lanza, G.
2023. Procedia CIRP, 120, 1125 – 1130. doi:10.1016/j.procir.2023.09.136
Beiner, S.; Kandler, M.; May, M. C.; Kinkel, S.; Lanza, G.; Richter, D.
2023. Production Processes and Product Evolution in the Age of Disruption – Proceedings of the 9th Changeable, Agile, Reconfigurable and Virtual Production Conference (CARV2023) and the 11th World Mass Customization & Personalization Conference (MCPC2023), Bologna, Italy, June 2023, 763 – 773, Springer International Publishing. doi:10.1007/978-3-031-34821-1_83
Dvorak, J.; Merforth, M.; Kandler, M.; Clever, F.; May, M. C.; Lanza, G.
2023. 13th Conference on Learning Factories (CLF 2023) Hrsg.: Hummel, Vera. doi:10.2139/ssrn.4471436
May, M. C.; Schäfer, L.; Frey, A.; Krahe, C.; Lanza, G.
2023. Procedia CIRP, 119, 944–949. doi:10.1016/j.procir.2023.02.172
Schäfer, L.; Treml, N.; May, M. C.; Lanza, G.
2023. Procedia CIRP, 119, 822–827. doi:10.1016/j.procir.2023.03.127
Frey, A. M.; May, M. C.; Lanza, G.
2023. Production Engineering, 12 (2), 263–277. doi:10.1007/s11740-022-01176-1
May, M. C.; Fang, Z.; Eitel, M. B. M.; Stricker, N.; Ghoshdastidar, D.; Lanza, G.
2023. Production Engineering, 17 (2), 211–222. doi:10.1007/s11740-022-01179-y
Kuhnle, A.; May, M. C.; Schäfer, L.; Lanza, G.
2022. International Journal of Production Research, 60 (19), 5812–5834. doi:10.1080/00207543.2021.1972179
Brützel, O.; Thiery, D.; May, M.; Lanza, G.
2022. ZWF Zeitschrift fuer Wirtschaftlichen Fabrikbetrieb, 117 (9), 591–596. doi:10.1515/zwf-2022-1106
Valet, A.; Altenmüller, T.; Waschneck, B.; May, M. C.; Kuhnle, A.; Lanza, G.
2022. Journal of Manufacturing Systems, 64, 518–534. doi:10.1016/j.jmsy.2022.07.016
Kandler, M.; Gabriel, P.; Schröttle, V.; May, M. C.; Lanza, G.
2022. Proceedings CPSL 2022 : Proceedings of the Conference on Production Systems and Logistics : CPSL 2022. Ed.: D. Herberger, 642–651, publish-Ing. doi:10.15488/12153
Overbeck, L.; Rose, A.; May, M.; Lanza, G.
2022. ZWF Zeitschrift fuer Wirtschaftlichen Fabrikbetrieb, 117 (4), 244–248. doi:10.1515/zwf-2022-1035
May, M. C.; Neidhöfer, J.; Körner, T.; Schäfer, L.; Lanza, G.
2022. Procedia CIRP, 10th CIRP Global Web Conference – Material Aspects of Manufacturing Processes, 115, 184–189. doi:10.1016/j.procir.2022.10.071
Kandler, M.; Dierolf, L.; Bender, M.; Schäfer, L.; May, M. C.; Lanza, G.
2022. Procedia CIRP, 10th CIRP Global Web Conference – Material Aspects of Manufacturing Processes, 115, 190–195. doi:10.1016/j.procir.2022.10.072
Kuhn, A. M.; May, M. C.; Liu, Y.; Kuhnle, A.; Tekouo, W.; Lanza, G.
2022. Production Engineering, 17 (3-4), 739–752. doi:10.1007/s11740-022-01160-9
Hofmann, C.; Liu, X.; May, M.; Lanza, G.
2022. Production Engineering, 17, 133–144. doi:10.1007/s11740-022-01152-9
Schäfer, L.; Frank, A.; May, M. C.; Lanza, G.
2022. Procedia CIRP, 107, 469–474. doi:10.1016/j.procir.2022.05.010
Krahe, C.; Marinov, M.; Schmutz, T.; Hermann, Y.; Bonny, M.; May, M.; Lanza, G.
2022. 32nd CIRP Design Conference (CIRP Design 2022) - Design in a changing world. Ed.: N. Anwer, 275–280, Elsevier. doi:10.1016/j.procir.2022.05.249
May, M. C.; Kiefer, L.; Kuhnle, A.; Lanza, G.
2022. Applied Sciences (Switzerland), 12 (3), Art.-Nr.: 1608. doi:10.3390/app12031608
Wurster, M.; Michel, M.; May, M. C.; Kuhnle, A.; Stricker, N.; Lanza, G.
2022. Journal of Intelligent Manufacturing, 33 (2), 575–591. doi:10.1007/s10845-021-01863-3
Kandler, M.; May, M. C.; Kurtz, J.; Kuhnle, A.; Lanza, G.
2022. Towards Sustainable Customization: Bridging Smart Products and Manufacturing Systems: Proceedings of the 8th Changeable, Agile, Reconfigurable and Virtual Production Conference (CARV2021) and the 10th World Mass Customization & Personalization Conference (MCPC2021), Aalborg, Denmark, October/November 2021. Ed.: A.-L. Andersen, 738–745, Springer. doi:10.1007/978-3-030-90700-6_84
May, M. C.; Schmidt, S.; Kuhnle, A.; Stricker, N.; Lanza, G.
2021. Procedia CIRP, 96, 45–50. doi:10.1016/j.procir.2021.01.050
May, M. C.; Kiefer, L.; Kuhnle, A.; Stricker, N.; Lanza, G.
2021. Procedia CIRP, 96, 3–8. doi:10.1016/j.procir.2021.01.043
Fleischer, J.; Lanza, G.; Wirth, F.; Gönnheimer, P.; Peukert, S.; May, M.; Hausmann, L.; Fraider, F.; Netzer, M.; Oexle, F.; Silbernagel, R.; Overbeck, L.
2021. VDMA Antriebstechnik
May, M. C.; Albers, A.; Fischer, M. D.; Mayerhofer, F.; Schäfer, L.; Lanza, G.
2021. Forecasting, 2021 (2), 322–338. doi:10.3390/forecast3020021
May, M. C.; Behnen, L.; Holzer, A.; Kuhnle, A.; Lanza, G.
2021. 9th CIRP Global Web Conference – Sustainable, resilient, and agile manufacturing and service operations : Lessons from COVID-19. Ed.: K. Medini, 55–60, Elsevier. doi:10.1016/j.procir.2021.10.008
Overbeck, L.; Hugues, A.; May, M. C.; Kuhnle, A.; Lanza, G.
2021. 9th CIRP Global Web Conference – Sustainable, resilient, and agile manufacturing and service operations : Lessons from COVID-19. Ed.: K. Medini, 170–175, Elsevier. doi:10.1016/j.procir.2021.10.027
May, M. C.; Overbeck, L.; Wurster, M.; Kuhnle, A.; Lanza, G.
2021. Procedia CIRP, 99, 27–32. doi:10.1016/j.procir.2021.03.005
May, M. C.; Maucher, S.; Holzer, A.; Kuhnle, A.; Lanza, G.
2021. Procedia CIRP, 100, 49–54. doi:10.1016/j.procir.2021.05.008
Kapp, V.; May, M. C.; Lanza, G.; Wuest, T.
2020. Journal of Manufacturing and Materials Processing, 4 (3), 88. doi:10.3390/jmmp4030088
Kain, M.; Nadimpalli, V. K.; Miqueo, A.; May, M. C.; Yagüe-Fabra, J. A.; Häfner, B.; Pedersen, D. B.; Calaon, M.; Tosello, G.
2020. Proceedings of the 20th International Conference of the European Society for Precision Engineering and Nanotechnology, EUSPEN 2020. Ed.: R. K. Leach, 175–176, European Society for Precision Engineering and Nanotechnology (euspen)